2025 will be a defining year for AI, shifting from generalized applications to enterprise-focused solutions. Businesses will go beyond generic-style content, and refine their strategies to target specific use cases that deliver measurable results. This will really bring home that AI development is only as good as the training data it is trained on. It is increasingly important to recognize that crowdsourcing data for AI training enables organizations to gather diverse, high-quality data at scale, fuelling robust AI models.
LXT is a good example of a platform that facilitates the collection of varied datasets (text, speech, images) tailored to specific AI development needs, and we have chosen LXT to provide us with several case study examples in this blog.
The business was founded in Canada in 2010, and remains headquartered in Toronto. LXT got its start providing high-quality Arabic data for a Big Tech client. Since its acquisition in December 2024 of the freelance gig-economy platform clickworker, LXT can claim it has over 20 years’ experience of AI development, working with more than 7 million people in 145 countries, and in over 1,000 languages and dialects.
1. Crowdsourcing for Scalability and Speed
Crowdsourcing allows businesses to gather and label data faster than traditional in-house methods. This accelerates AI training cycles and brings solutions to market more quickly.
Data provider companies, including LXT, have reduced time-to-market using crowdsourced data annotation significantly. Their case studies include a Top 10 global technology company that wanted to extend support for its keyboard to multiple countries to drive customer adoption. The keyboard needed to support a large variety of languages and dialects, including rare languages that are only spoken by a few thousand people. LXT developed a crowdsourced data collection program that involved collecting data in almost 60 languages. Early results impressed the client enough to expand the program to cover 120 languages. The initial plan had allowed 12 months to complete the program. Researching all 120 languages was achieved in six months.
In another example, a top ten global technology company wanted to fine tune the foundational Large Language Models that power its Generative AI solution in 50 languages to reach a wide range of global users. Its goal was to ensure that the solution’s output was evaluated by a diverse pool of human contributors for accuracy, clarity and bias.
This was a very time-sensitive project, and a large crowd of contributors needed to be onboarded very quickly to perform the rating and evaluation tasks whilst meeting very tight internal deadlines. LXT successfully qualified and onboarded 4,000 contributors across all 50 languages in one week.
2. Diversity and Bias Mitigation in AI Models
Crowdsourcing leverages diverse global contributors, minimizing biases in AI datasets and ensuring inclusivity in AI applications.
A top 10 global technology company wanted to enhance the capability and user experience of its smartphones, keyboards and apps by providing emoji suggestions in 60 languages. LXT knew that it would require highly creative freelancers who could provide detailed descriptions of each emoji in their native language. A robust review process ensured that high quality standards were achieved before delivering the data to the client. The emoji transcriptions in the 60 target languages were achieved in four months, ahead of schedule.
This highlights crowdsourcing’s ability to generate data from a variety of demographics, languages, and regions to train unbiased AI systems.
3. Cost Benefits of Crowdsourced AI Data
Building an in-house data annotation team is expensive and resource-intensive. Crowdsourcing provides a cost-effective alternative for AI training by outsourcing data labelling to qualified contributors. They are usually remunerated on the basis of the results they deliver, not for the time they occupy a desk and chair as a salaried employee.
Companies using crowdsourcing platforms can therefore scale data collection efforts without incurring significant overhead costs.
4. Applications Across Industries
Crowdsourcing can fuel AI innovation across various sectors, including:
- Retail: Personalization and recommendation systems.
- Healthcare: Medical image annotation for diagnostic AI.
- Finance: Fraud detection and risk assessment models.
- Technology: Chatbots, virtual assistants, and voice recognition systems.
L’Oréal, for example, is a major beauty and cosmetics company that increasingly leverages user-generated content (UGC) across social media platforms like Instagram, TikTok, and YouTube. This vast amount of data includes product reviews, tutorials, “get ready with me” videos, and images showcasing makeup looks and skincare routines. This UGC acts as a massive, real-time dataset reflecting consumer preferences, application techniques, skin concerns, and trending styles. Deliveries of product samples for the influencers to showcase to their followers also helps to generate brand loyalty.
While L’Oréal has been using AI for some time, the scale and sophistication of leveraging UGC for these purposes have significantly increased recently with advancements in AI’s ability to process and understand complex, unstructured data. The focus on authentic user experiences reflected in UGC is a growing trend in retail AI. Whilst being a great example, L’Oréal is not an LXT client.
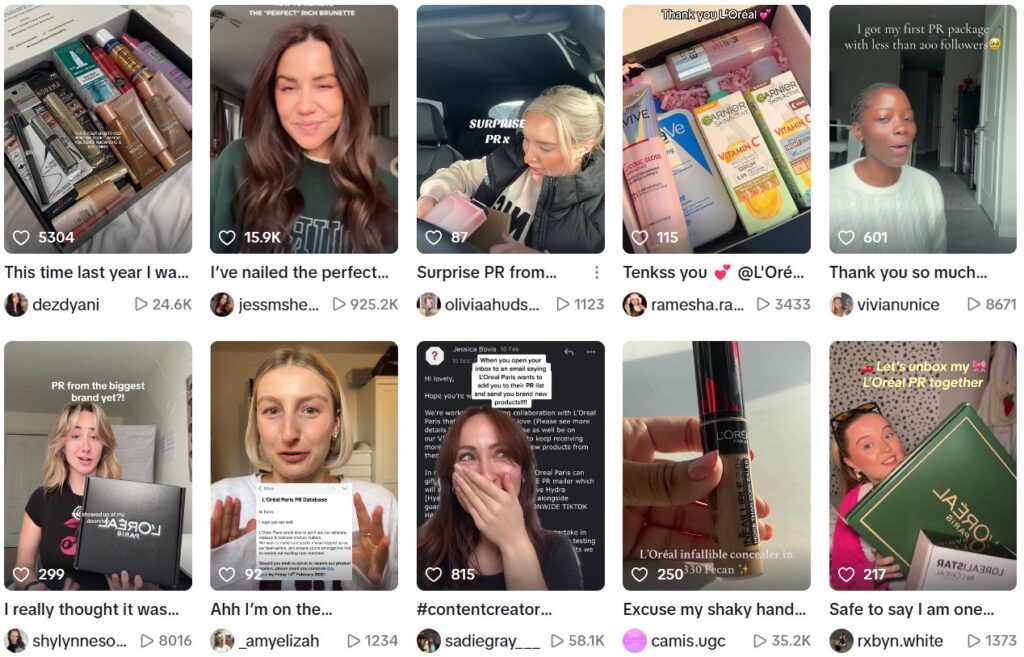
L’Oréal works with many social media influencers to crowdsource user generated content that supports AI training. Image source: TikTok
5. Simplifying the Crowdsourcing Workflow
Platforms like LXT streamline the crowdsourcing process, from data collection to annotation and validation, ensuring accuracy and efficiency. The acquisition of clickworker, one of the largest global providers of crowdsourced data, will allow the platform to integrate and expand to deliver top quality AI data solutions, with quality control measures and seamless integration with AI pipelines.
One of LXT’s clients, a leading provider of AI-powered visual assessment solutions, needed to collect large volumes of 360 degree walk around videos of vehicles to enhance its insurance assessment solution, as well as to develop a new offering related to automated vehicle condition reports. The company had worked with other AI data providers for video data collection but experienced a variety of quality issues including low resolution, low lighting, file mismatches, camera orientation problems and more.
The client originally required 1,000 videos. However, after 10 days the quality of the content that LXT was generating was high enough for the pilot to quickly and easily scale to a full program across 48 countries. LXT went on to collect 50,000 AI training videos in just five months, while maintaining the quality standards from the pilot phase.
6. Human-AI Collaboration in Crowdsourcing
Crowdsourcing is not just about human effort, but also how AI tools enhance the accuracy and efficiency of data annotation, and vice versa.
LXT uses AI-driven tools alongside human contributors – AI with HI – to ensure consistent, high-quality results. Its Reinforcement Learning from Human Feedback (RLHF) is critical to building responsible and explainable generative AI solutions. A key point in the RLHF process is to obtain data that can be used for fine-tuning generative AI models. With RLHF, a curated group of human contributors evaluates the output of generative AI solutions, providing human oversight to ensure that the machine learning models used to train these solutions deliver non-offensive, accurate and unbiased results.
7. Tackling Specific Challenges in AI Development
Crowdsourcing addresses common AI challenges, such as lack of localized data, language-specific datasets, or underrepresented demographics.
LXT’s network of over 7 million contributors enables it to specialize in niche data needs, such as rare languages or specific industry contexts. LXT supports data collection and annotation in more than 750 language locales and has recently seen an increase in demand for Canadian French. The company now operates four ISO27001 and PCI DSS compliant locations in Canada, as well as one in Egypt. These facilities support customers that require the utmost security to meet stringent standards set by the General Data Protection Regulation (GDPR) and Healthcare Insurance Portability and Accountability Act (HIPAA), among others.
8. Ethical Crowdsourcing Practices in AI Development
Ethical considerations are vital in crowdsourcing. Fair compensation, data privacy, and contributor safety are essential for responsible AI development, and to retain the loyalty and integrity of contributors.
Summary
The article discusses the pivotal role of crowdsourcing in providing scalable, high-quality training data for AI development in 2025, as enterprises shift toward specialized AI solutions. It highlights LXT, a Toronto-based company founded in 2010, which, after acquiring clickworker in 2024, leverages a global network of over 7 million contributors across 145 countries to deliver diverse datasets in over 750 languages. Key points include:
- Scalability and Speed: Crowdsourcing accelerates AI training by enabling rapid data collection and annotation. Case studies show a tech company expanding a keyboard language support program from 60 to 120 languages in six months and onboarding 4,000 contributors in one week for a generative AI project in 50 languages.
- Diversity and Bias Mitigation: Crowdsourcing ensures inclusive AI by sourcing data from varied demographics, reducing biases. For instance, LXT supported emoji suggestions in 60 languages, completed in four months.
- Cost Efficiency: Crowdsourcing reduces costs compared to in-house data annotation, as contributors are paid per task, not salaried.
- Industry Applications: Crowdsourced data fuels AI in retail (e.g., L’Oréal’s use of user-generated content for personalization), healthcare (medical image annotation), finance (fraud detection), and technology (chatbots, voice recognition).
- Streamlined Workflow: Enhanced by the acquisition of clickworker, LXT simplifies data collection, annotation, and validation. A client was confident to scale from an initial sample of 1,000 vbehicle videos to 50,000 in five months for insurance assessments, maintaining high quality.
- Human-AI Collaboration: Integration of AI tools with human oversight, using LXT’s Reinforcement Learning from Human Feedback (RLHF), ensures accurate, unbiased generative AI outputs.
- Addressing Niche Needs: LXT has a large enough network of contributors to tackle challenges like rare language data or industry-specific needs.
- Ethical Practices: Fair pay, data privacy, and contributor safety are emphasized for responsible AI development.
Overall, crowdsourcing, exemplified by LXT, is critical for delivering diverse, cost-effective, and ethically sourced data to drive advanced AI solutions across industries.
0 Comments